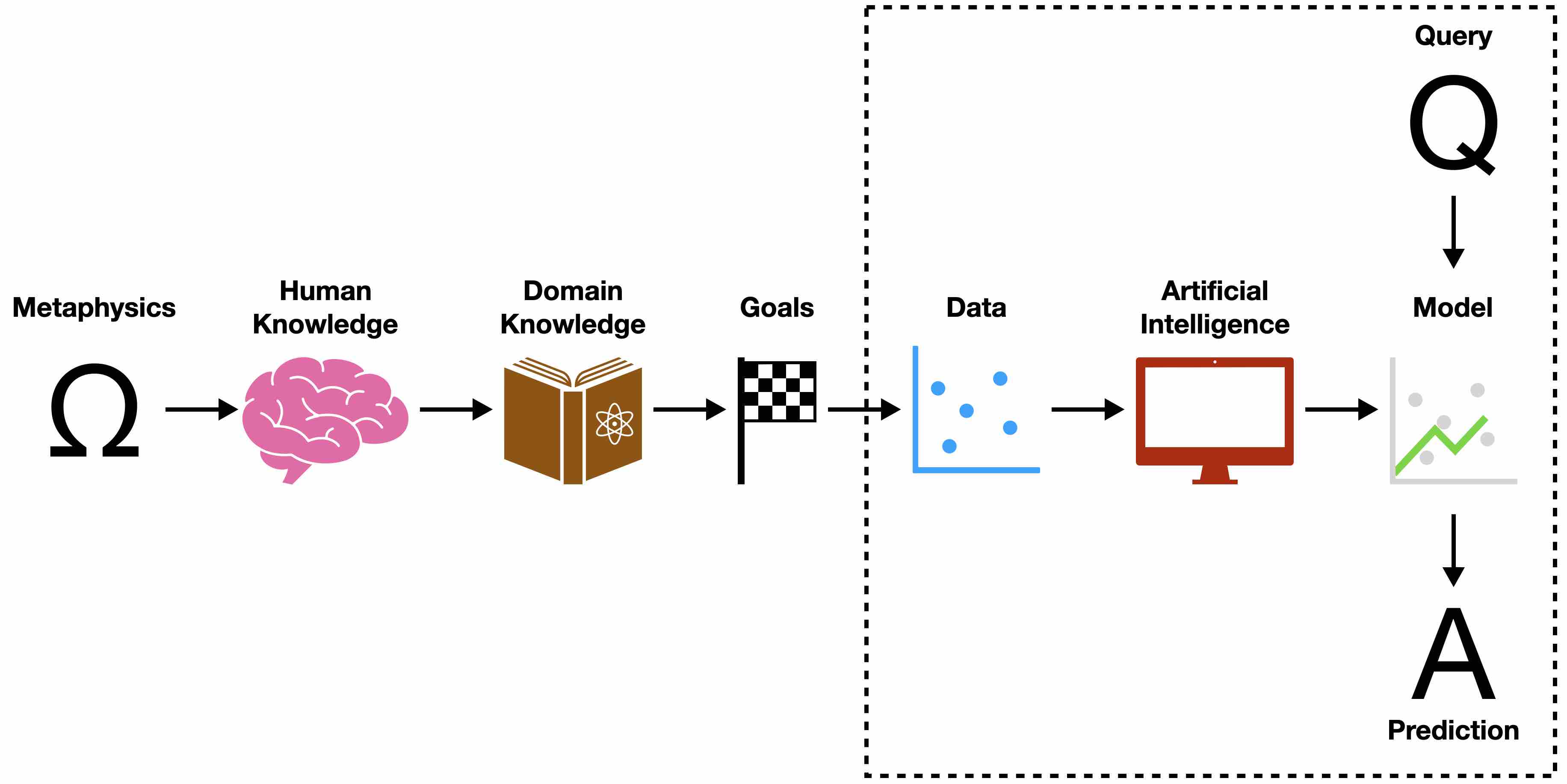
Informed Machine Learning
Auton Lab research frequently involves ways to incorporate expert knowledge into AI systems. We recognize that simply studying the data, model, and predictions is an unnecessarily limiting perspective because these factors are informed by upstream conditions, knowledge, and design choices. Figuring out how to incorporate this upstream knowledge into AI systems represents a huge opportunity to guide AI to learning data-driven policy that also respects common sense norms. Work in this context ranges from research on how to effectively have experts label vast amounts of data, to incorporating feedback in active learning frameworks, to formal verification of model adherence to domain-specific constraints and design specifications. We take AI outside the cozy spot of data-driven approach. Standard AI relies primarily on what can be learned from data, however, data is just a limited projection of reality. Auton lab is working on multiple exciting avenues to make AI and ML smarter.